Privacy and Fairness
As data science becomes pervasive across many areas of society, and as it is increasingly used to aid decision-making in sensitive domains, it becomes crucial to protect individuals by guaranteeing privacy and fairness. The investigators propose to research the theoretical foundations to providing such guarantees and to surface inherent limitations.
Learning on Social Graphs
Many of the fundamental questions in applying data science to the interactions between individuals and larger social systems involve the social networks that underpin the connections between individuals. The researchers will develop new techniques for understanding both the structure of these networks and the processes that take place within them.
Learning to Intervene
Data-driven approaches to learning good interventions (including policies, recommendations, and treatments) inspire challenging questions about the foundations of sequential experimental design, counterfactual reasoning, and causal inference.
Uncertainty Quantification
Quantifying uncertainty about specific predictions or conclusions represents a key need in data science, especially when applied to decision-making with potential consequences to human subjects. The researchers will develop statistical tools and theoretical guarantees to assess the uncertainties of predictions made by popular algorithms in data science.
Deep Learning
Deep Learning algorithms have made impressive advances in practical settings. Although their basic building blocks are well understood, there is still ambiguity about what they learn and why they generalize so well. There are indications that they may learn data manifolds and that the type of optimization algorithm influences generalization.
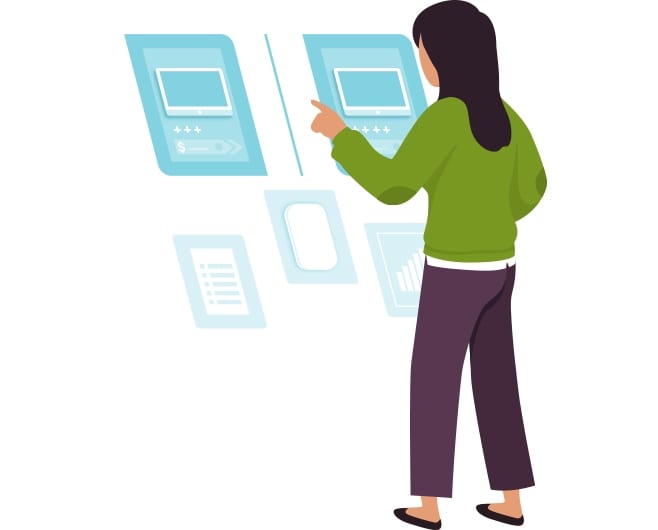